AI agents in the wild
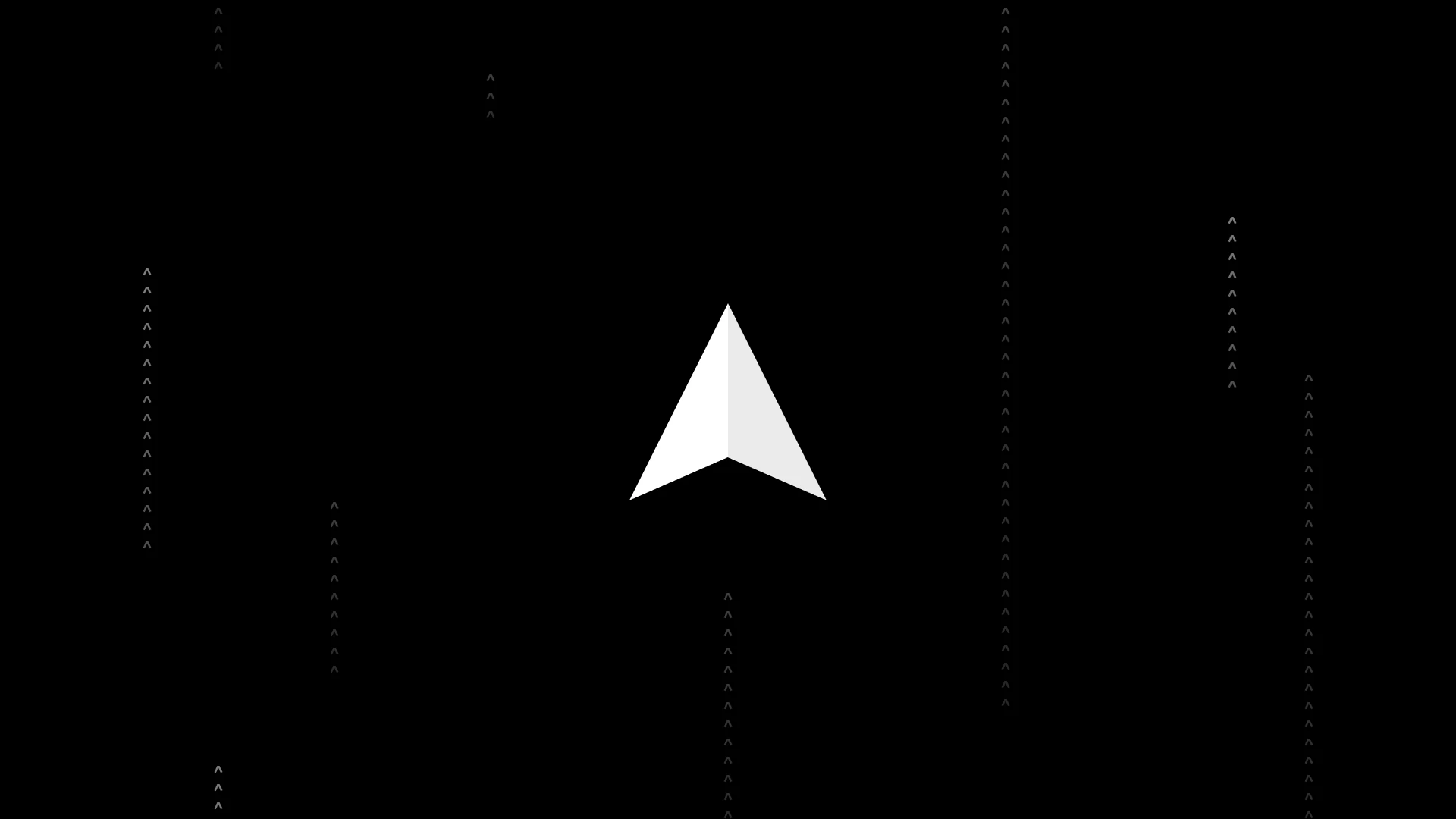
They are all over here and more of them are coming soon.
In the last months, AI agents have gained huge popularity - AutoGPT has reached 140,000 stars on GitHub and we’re seeing new companies being started on an almost weekly basis. Ranging from agents reviewing code (Sweep AI) to virtual personal assistants like Lindy.
We mapped the AI agents landscape and explored over 50 agents, which we put together into a list. Here are our conclusions about the current state of the ecosystem.
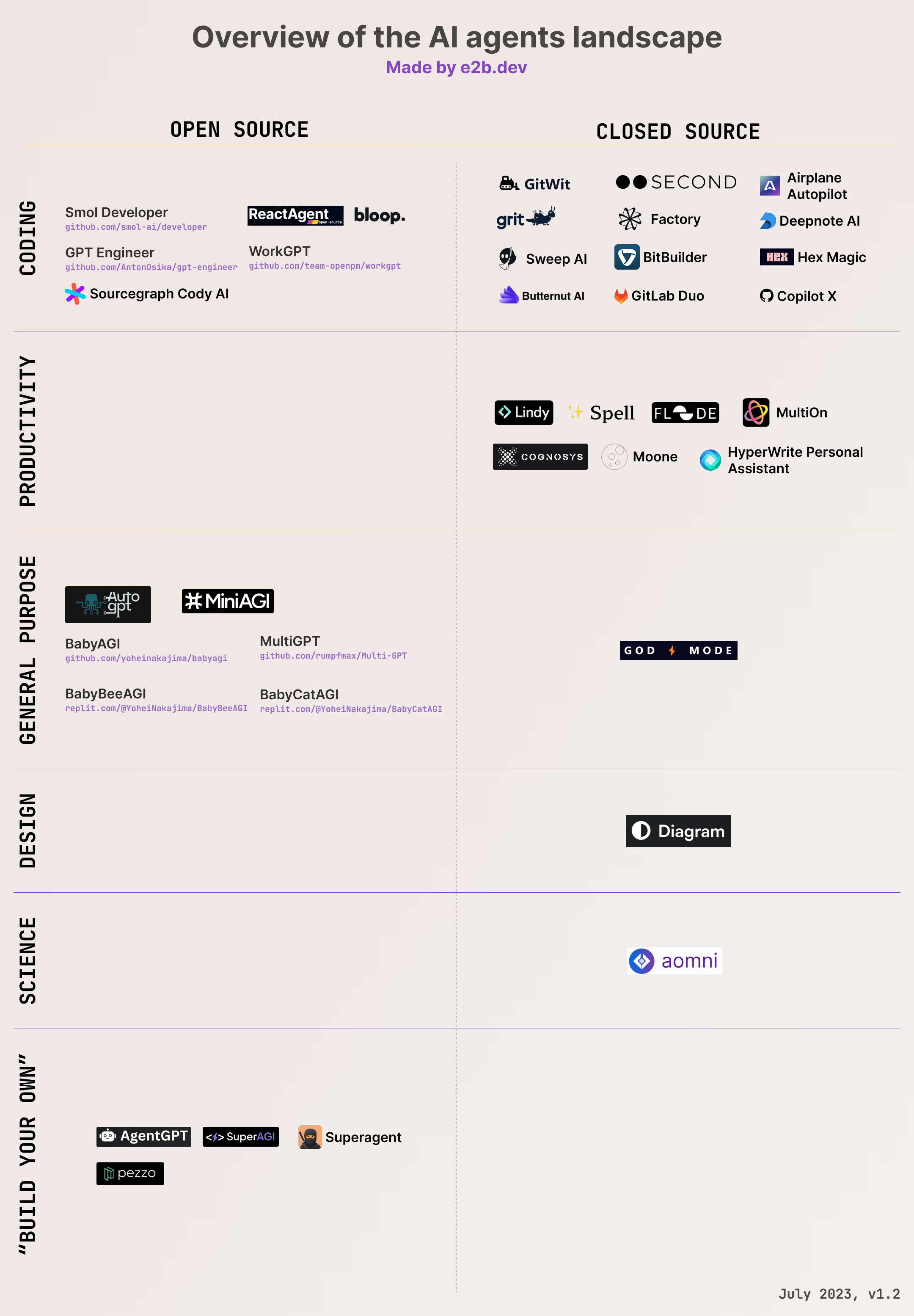
What is an AI autonomous agent?
Developers and founders have been eager to contribute to the agents’ list with various agent-ish projects. We have to ask the prerequisite question though. How do you define an autonomous AI agent? How would one differentiate between an AI agent and LLM-based chatbot, for example?
Agents use both short-term memory (in-context learning) and long-term memory (retrieval of information from an external vector store), have the capability to plan, break down objectives into smaller tasks by “thinking” step-by-step, and reflect on their own performance. Moreover, a lot of agents can use tools, such as scraping websites, using Google Calendar, or running commands in the developer’s terminal. Implementing tool usage has been made easier for developers by the recent OpenAI's explicit support for functions.
We aimed to include projects and companies based on this empirical definition.
Five key learnings
- Among 56 projects, we observed an approximately 50:50 ratio of open source vs closed source projects.
- The closed source projects are generally better at communicating use cases for their agent.
- The most popular use case is coding assistants, followed by productivity assistants, and general purpose agents.
- There’s a high number of no-code/low-code platforms for building custom agents. Users of these platforms must be somewhat familiar with technicalities of LLMs to be able to use them efficiently.
- Developers of agents most often struggle with testing/evaluating, debugging, monitoring, and identifying at what step their agent broke and why.
What's next
The hype around AI agents is strong, however, as much as AI agents are exciting and promising there’s still a lot of challenges, especially around their reliability. We think AI agents are on a path to become the future of software and become common the same way for example websites are common nowadays. However, to get there, there needs to be first better tooling for developers. Especially tooling that will help developers make their agents more reliable and give developers bigger confidence their agents are working as intended.